Supervised Fine Tuning for Gemini: A Best Practices Guide
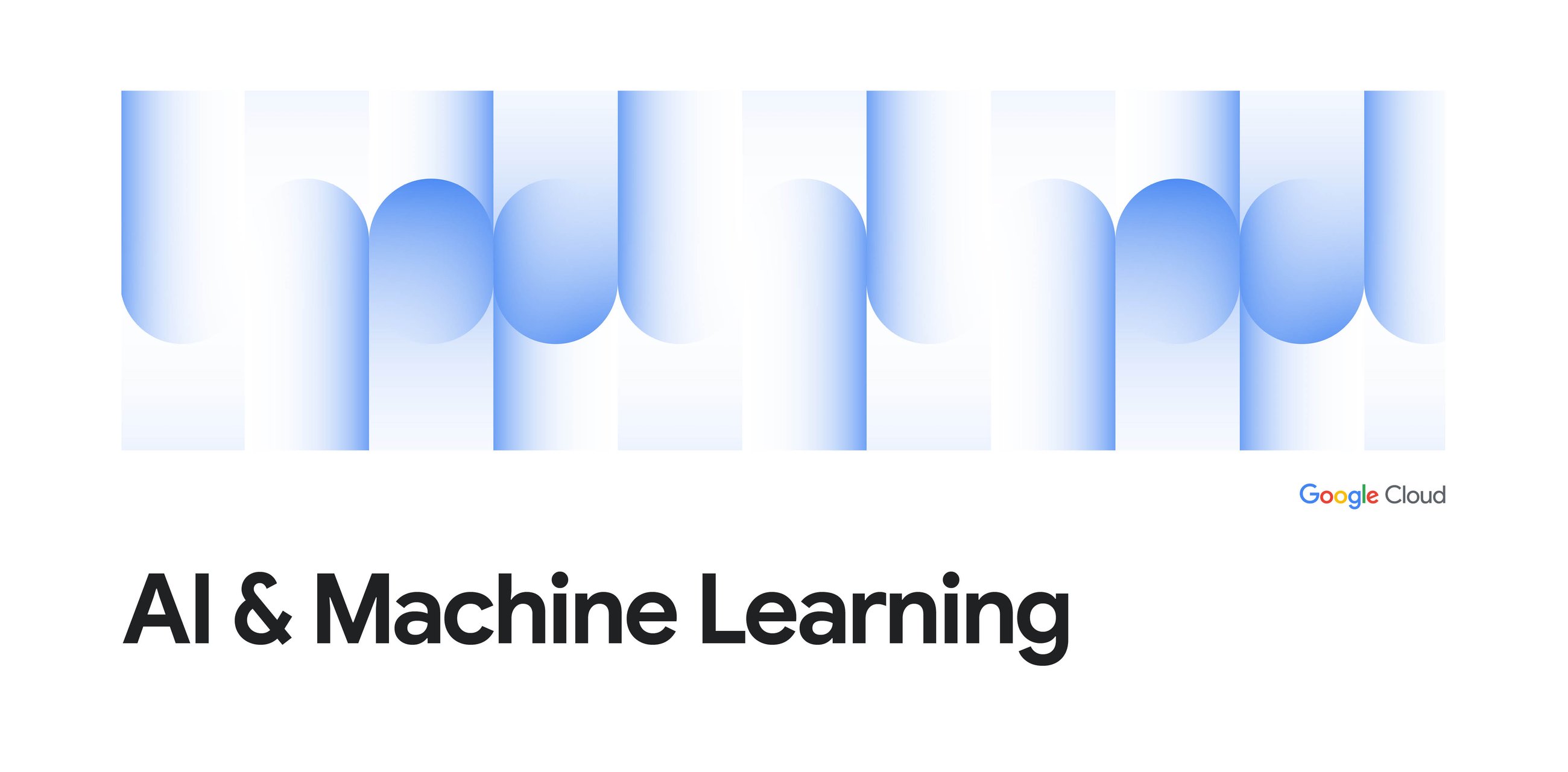
Explore effective strategies for supervised fine-tuning of the Gemini model to enhance performance on specialized business tasks.
Supervised fine-tuning (SFT) is a game-changer in optimizing foundation models like Gemini for specific business applications. While these models bring revolutionary capabilities, they sometimes fall short in addressing structured tasks effectively—such as providing overly lengthy answers or missing key points in summaries. SFT offers a structured approach to refine these models, enabling them to deliver precise performance tailored to unique tasks, domains, and stylistic nuances crucial for business settings.
When developers implement SFT, several best practices can significantly enhance the process. Initially, selecting the right model version to align with specific needs is vital, such as opting for Gemini 1.5 Pro for high accuracy or Gemini 1.5 Flash for cost-efficient, low-latency performance. Properly crafting a high-quality dataset is equally important; developers should focus on producing diverse, relevant, and accurate examples to optimize training efficiency and effectiveness. Incorporating detailed instructions into the dataset, recognizing crucial hyperparameters, and employing a dedicated validation dataset further refine the training, ensuring that each adjustment is data-driven and purposefully aligned with production requirements. By maintaining a keen focus on these elements, practitioners can achieve superior results with their fine-tuning efforts.